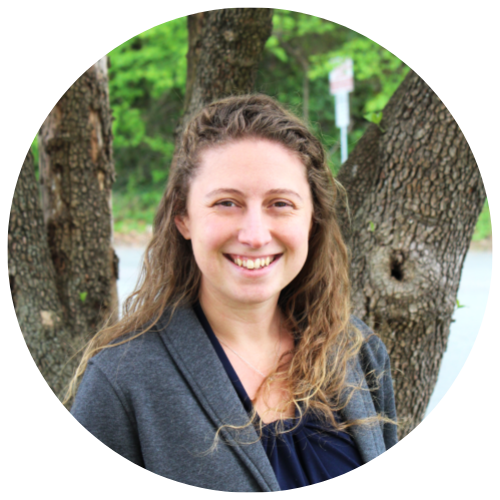
The corrosion of aviation and missiles costs the U.S. Department of Defense more than $10 billion annually and accounts for nearly 25% of all maintenance.1 Traditionally, aircraft corrosion prevention and control is done using calendar-based inspection and maintenance intervals. These methods may be overly conservative to avoid widespread corrosion degradation but may still miss corrosion of aircraft that have experienced severe service environments.
Transitioning instead to a usage-based maintenance schedule, through individual asset tracking, would help decrease costly unscheduled maintenance and repair. Predictive models for severity tracking and assessment can be used to improve the efficiency of preventative corrosion maintenance to increase asset availability and decrease lifecycle cost.2
So how can we improve corrosion prediction on globally distributed, mobile assets incorporating the effects of environmental impacts such as proximity to the ocean, airborne contaminants, local weather events, and seasonal variations, to enable more timely and accurate corrosion maintenance?
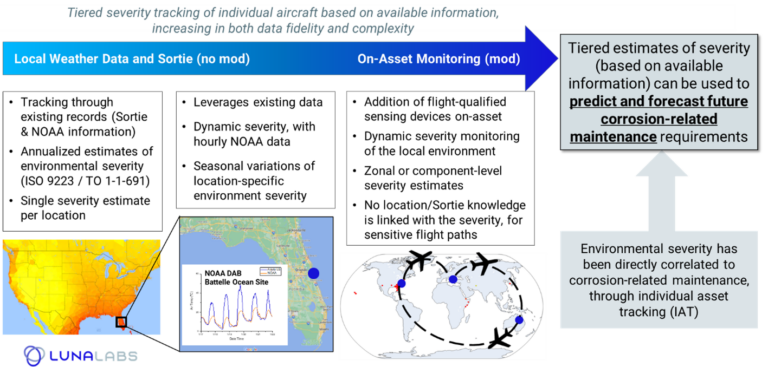
Using Tiered Models to Predict and Forecast Corrosion Severity
Our systems integration and corrosion products team is developing a series of tiered models to predict and forecast the corrosion severity based on available user data, ranging from rapid estimates with readily available data (annual averaged severity) to higher-fidelity predictions (hourly dynamic severity) for individual assets. To accomplish this approach, both virtual and on-aircraft monitoring are leveraged. The former represent corrosion predictions for an asset or location without the need for deployed measurements systems; using only environment models and transfer functions, the corrosivity can be mapped to a structure or location. Virtual monitoring of difficult-to-access locations can be used to supplement physical deployments of real-time monitoring. In this way, computational tools are used to complement deployed measurement systems, rather than replacing them.
The goal of each model within the tiered approach is to track corrosion severity and forecast future severity of individual aircraft throughout their lifecycle to enable fleet wide usage-based maintenance.
Aspects of this approach were included in the AMPP 2024 conference proceedings (see a summary of Luna Labs and Acuity presentations here) where weather parameters and local Acuity measurements at four coastal locations across the US were used to train a series of data-driven machine learning models. An advantage of the tiered approach is that models can be developed in parallel and subdivided into modules for integration with enterprise management systems.
“This initial tiered model approach performed best when dynamic salt accumulation or local environment measurements were incorporated,” explained Dr. Rebecca Marshall, Corrosion Scientist at Luna Labs who is leading this initiative. “The study showed that understanding environmental parameters can lead to forecasted severity and corrosion-related maintenance manhours for a given asset to enable informed maintenance schedule intervals, reduce costs, and improve asset availability.”
“Our models become more accurate as we increase the amount of data,” stated Pierre Morel, Director of the Systems Group at Luna Labs. “NOAA data on its own is helpful but has limited precision. When we add local environmental data plus flight data, we get more precision. The ultimate goal is to use all this data plus onboard corrosion sensors for accurate, precise predictions of corrosion severity on each individual asset.”
Additional information related to this work:
References
- Herzberg, E., A. Morris, A. Kelly, R. Stroh, L. Rutledge, and N. O’Meara, (2018): p. 68.
- Parker, D. & Bellocchio, A. Lt. Col. The Maintenance Forecast. Army AL&T pp.77–81 (2022).
If you have an asset that needs to be monitored, contact us for information about Acuity Corrosion Technologies.
If you are interested in a prediction model for your own application, let us know.